New study finds way to spot AI hallucinations
AI models can ‘hallucinate’ or make up an answer when they are uncertain about how to answer a query.
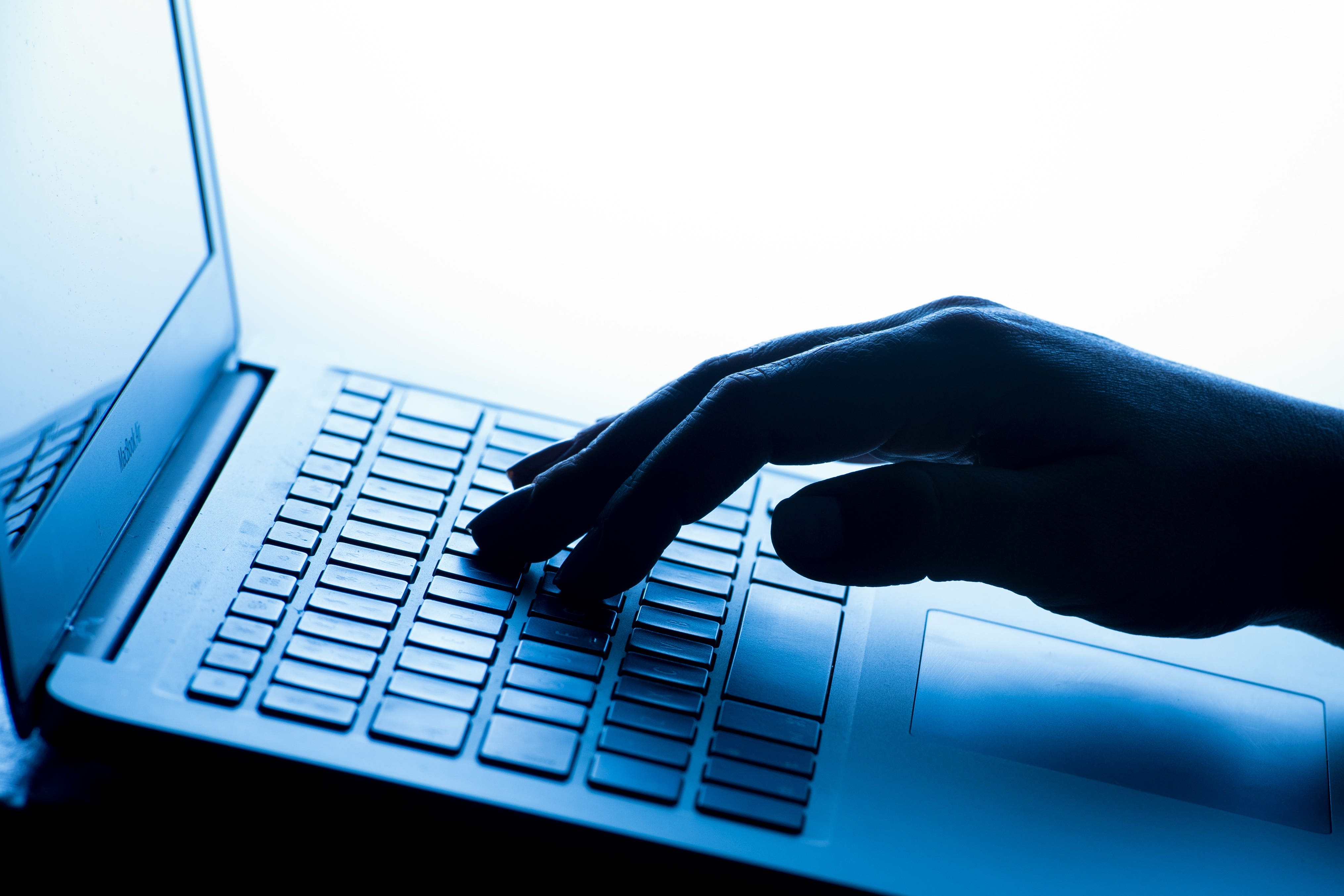
Researchers have found a new method which could be used to help spot when generative AI is likely to “hallucinate” – where it invents facts because it does not know the answer to a query – and help prevent such incidents occurring.
A new study by a team from the University of Oxford developed a statistical model which could identify when a question asked of a large language model (LLM) used to power a generative AI chatbot was likely to produce an incorrect answer.
Their research has been published in the journal Nature.
Hallucinations have been identified as a key concern around generative AI models, as the advanced nature of the technology and their conversational ability means they are able to pass up made up information as fact in order to respond to a query.
At a time when more students are turning to generative AI tools to help with research and to complete assignments – tasks many models are being marketed as helpful at – many industry experts and AI scientists are calling for more action to be taken to combat AI hallucinations, in particular when it comes to medical or legal queries.
The researchers at the University of Oxford said their research had found a way of telling the difference between when a model is certain about an answer or just making something up.
Study author Dr Sebastian Farquhar said: “LLMs are highly capable of saying the same thing in many different ways, which can make it difficult to tell when they are certain about an answer and when they are literally just making something up.
“With previous approaches, it wasn’t possible to tell the difference between a model being uncertain about what to say versus being uncertain about how to say it. But our new method overcomes this.”
But Dr Farquhar said there was further work to do on ironing out the errors AI models can make.
“Semantic uncertainty helps with specific reliability problems, but this is only part of the story,” he said.
“If an LLM makes consistent mistakes, this new method won’t catch that. The most dangerous failures of AI come when a system does something bad but is confident and systematic.
“There is still a lot of work to do.”
Subscribe to Independent Premium to bookmark this article
Want to bookmark your favourite articles and stories to read or reference later? Start your Independent Premium subscription today.