The secret bias hidden in mortgage-approval algorithms
An investigation by The Markup has found that lenders in 2019 were more likely to deny home loans to people of color than to white people with similar financial characteristics
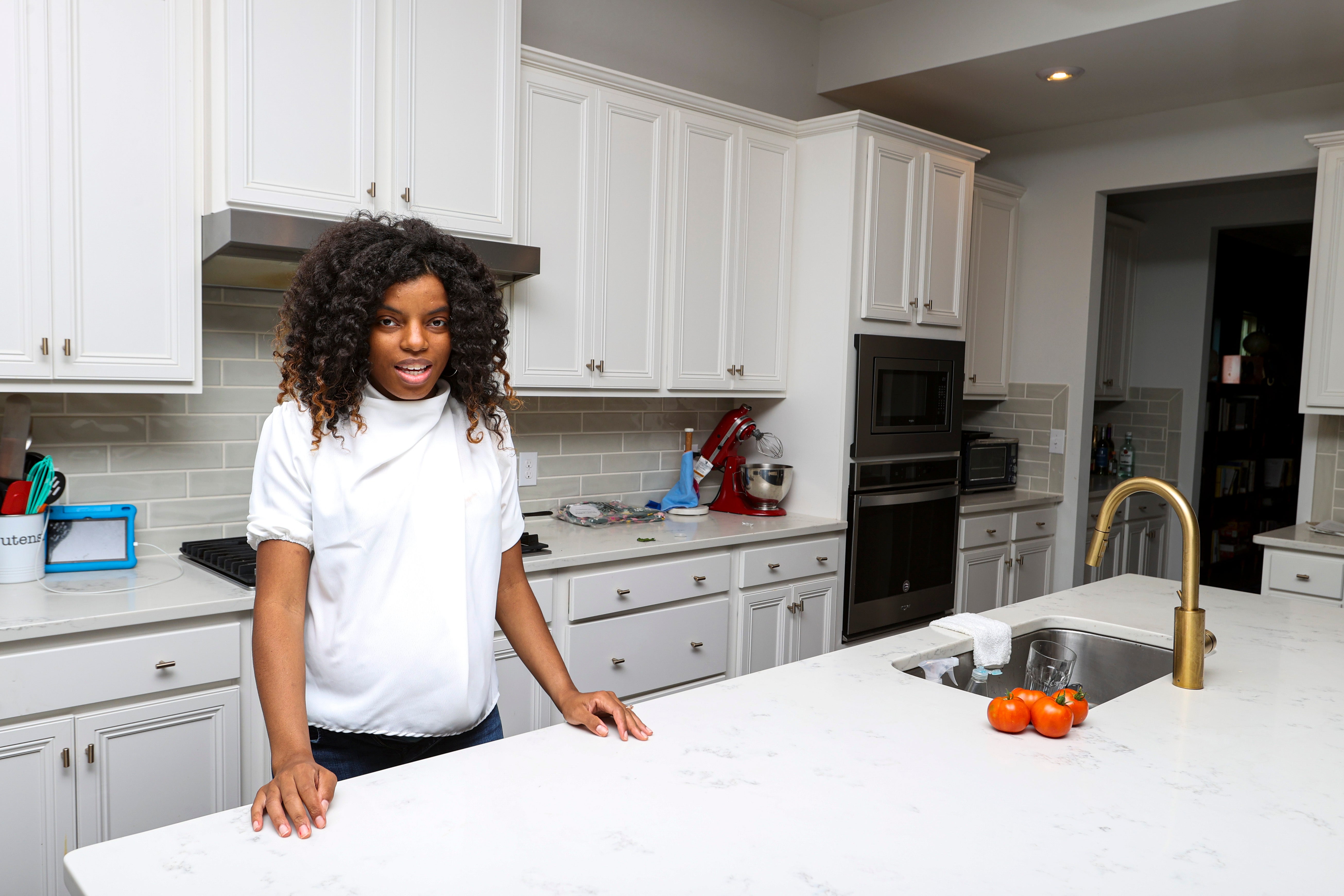
The new four-bedroom house in Charlotte North Carolina was Crystal Marie and Eskias McDaniels’ personal American dream, the reason they had moved there from pricey Los Angeles
A lush, long lawn, 2,700 square feet of living space, gleaming kitchen, and a neighborhood pool and playground for their son, Nazret. All for $375,000.
Pre-qualifying for the mortgage was a breeze: They had high credit scores, earned roughly six figures each and had saved more they would need for the down payment.
But two days before they were supposed to sign, in August 2019, the loan officer called Crystal Marie with bad news: The deal wasn’t going to close.
“It seemed like it was getting rejected by an algorithm,” she said, “and then there was a person who could step in and decide to override that or not.”
She was told she didn’t qualify because she was a contractor, not a full-time employee — even though her co-workers were contractors, too. And they had mortgages.
Crystal Marie’s co-workers are white. She and Eskias are Black.
“I think it would be really naive for someone like myself to not consider that race played a role in the process,” she said.
An investigation by The Markup has found that lenders in 2019 were more likely to deny home loans to people of color than to white people with similar financial characteristics — even when we controlled for newly available financial factors that the mortgage industry has in the past said would explain racial disparities in lending.
—
This story was reported by The Markup, and the story and data were distributed by The Associated Press.
—
Holding 17 different factors steady in a complex statistical analysis of more than 2 million conventional mortgage applications for home purchases reported to the government, we found that, in comparison to similar white applicants, lenders were:
● 80% more likely to reject Black applicants
● 70% more likely to deny Native American applicants
● 50% more likely to turn down Asian/Pacific Islander applicants
● 40% more likely to reject Latino applicants
These are national rates.
When we examined cities and towns individually, we found disparities in 90 metros spanning every region of the country. Lenders were 150% more likely to reject Black applicants in Chicago than similar white applicants there. Lenders were more than 200% more likely to reject Latino applicants than white applicants in Waco, Texas, and to reject Asian and Pacific Islander applicants than white ones in Port St. Lucie, Florida. And they were 110% more likely to deny Native American applicants in Minneapolis.
“Lenders used to tell us, ‘It’s because you don’t have the lending profiles; the ethno-racial differences would go away if you had them,’” said José Loya, assistant professor of urban planning at UCLA who has studied public mortgage data extensively and reviewed our methodology. “Your work shows that’s not true.”
The American Bankers Association, The Mortgage Bankers Association, The Community Home Lenders Association, and The Credit Union National Association all criticized the analysis.
In written statements, the ABA and MBA dismissed our findings for failing to include credit scores or government loans, which are mortgages guaranteed by the Federal Housing Administration, Department of Veterans Affairs and others.
Government loans have different thresholds for approval, which bring people into the market who wouldn’t otherwise qualify, but generally cost buyers more. Even the Federal Reserve and Consumer Financial Protection Bureau the agency that releases mortgage data, separate conventional and government loans in their research on lending disparities.
It was impossible for us to include credit scores in our analysis because the CFPB strips them from the public version of the data — in part due to the mortgage industry’s lobbying, citing borrower privacy.
While home lending decisions are officially made by loan officers at each institution, they are largely driven by software, most of it mandated by a pair of quasi-governmental agencies.
Freddie Mac and Fannie Mae were founded by the federal government to spur homeownership and now buy about half of all mortgages in America. As a result, they essentially set the rules from the very beginning of the mortgage-approval process.
They require lenders to use a particular credit scoring algorithm, “Classic FICO,” to determine whether an applicant meets the minimum threshold to be considered for a conventional mortgage in the first place, currently a score of 620.
Launched more than 15 years ago based on data from the 1990s, Classic FICO is widely considered detrimental to people of color because it rewards traditional credit, to which they have less access than white Americans. It doesn’t consider, among other things, on-time payments for rent, utilities, and cellphone bills — but will lower people’s scores if they get behind on those bills and sent to debt collectors. Unlike more recent models, it penalizes people for past medical debt after it’s been paid.
Yet Fannie and Freddie have resisted a stream of plaintive requests since 2014 from advocates, the mortgage and housing industries, and Congress to allow a newer model. They did not respond to questions about why.
The approval process also requires a green light by Fannie or Freddie’s automated underwriting software. Not even their regulator, the FHFA, knows exactly how they decide, but some of the factors the companies say their programs consider can affect people differently depending on their race or ethnicity, researchers have found.
For instance, traditional banks are less likely than payday loan sellers to place branches in neighborhoods populated mainly by people of color. Payday lenders don’t report timely payments, so they can only damage credit.
Gig workers who are people of color are more likely to report those jobs as their primary source of income, rather than a side hustle, than white gig workers. This can make their income seem more risky.
Considering an applicant’s assets beyond the down payment, which lenders call “reserves,” can cause particular problems for people of color. Largely due to intergenerational wealth and past racist policies, the typical white family in America today has eight times the wealth of a typical Black family, and five times the wealth of a Latino family. White families have larger savings accounts and stock portfolios than people of color.
The president of the trade group representing real estate appraisers recently acknowledged racial bias is prevalent in the industry, which sets property values, and launched new programs to combat bias.
“If the data that you’re putting in is based on historical discrimination,” said Aracely Panameño, director of Latino affairs for the Center for Responsible Lending, “then you’re basically cementing the discrimination at the other end.”
In written statements, Fannie said its software analyzes applications “without regard to race” and both Fannie and Freddie said their algorithms are routinely evaluated for compliance with fair lending laws, internally and by the FHFA and the Department of Housing and Urban Development. HUD said it has asked the pair to make changes as a result, but would not disclose the details.
Many large lenders also run applicants through their institutions’ own underwriting software. How those programs work is even more of a mystery; they are also proprietary.
Some fair lending advocates have begun to ask whether the value system in mortgage lending should be tweaked.
“As an industry, we need to think about, what are the less discriminatory alternatives, even if they are a valid predictor of risk,” said David Sanchez, a former FHFA policy analyst, who currently directs research and development at the nonprofit National Community Stabilization Trust. “Because if we let risk alone govern all of our decisions, we are going to end up in the exact same place we are now when it comes to racial equity in this country.”
Crystal Marie and Eskias McDaniels' lender denied race had anything to do with their denial. In an email, loanDepot vice president of communications Lori Wildrick said the company follows the law and expects “fair and equitable treatment” for every applicant.
The couple refused to give up after the loan officer told them the mortgage fell through and enlisted their real estate agent to help. Crystal Marie’s employer sent multiple emails vouching for her.
Around 8 p.m. on the night before the original closing date, Crystal Marie got an email from the lender: “You’re cleared to close.” She still doesn’t understand how she got to yes, but she was relieved and elated.
“It means so much to me, as a Black person,” said Crystal Marie, who said her family descended from slaves in neighboring South Carolina, “to own property in a place where not that many generations ago you were property.
“It’s meant so much.”
___
This story was reported by The Markup, and the story and data were distributed by The Associated Press.
Subscribe to Independent Premium to bookmark this article
Want to bookmark your favourite articles and stories to read or reference later? Start your Independent Premium subscription today.