New artificial intelligence can learn how to play vintage video games from scratch
The Deep Q-network has learned to play Space Invaders and Breakout
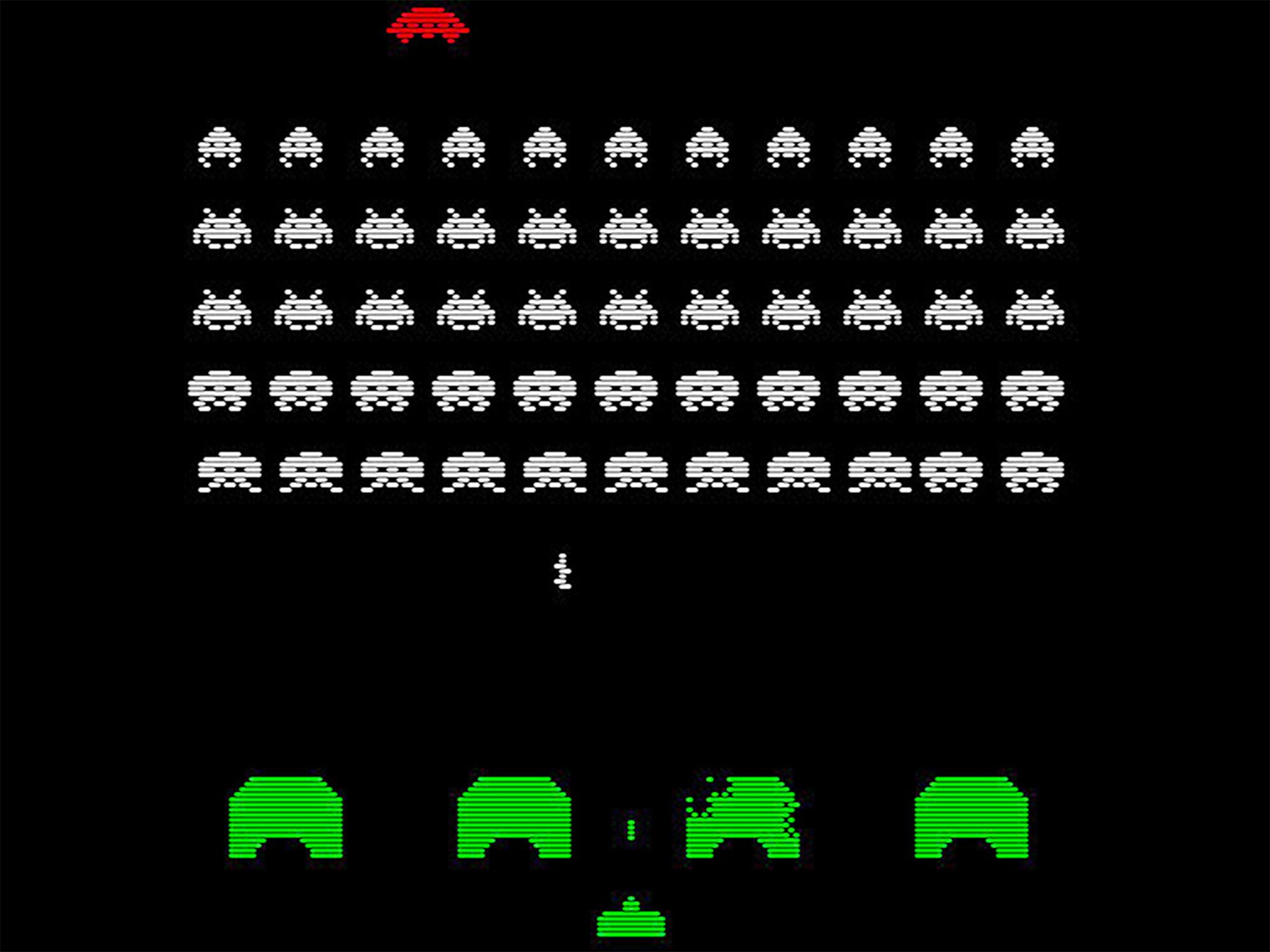
Your support helps us to tell the story
From reproductive rights to climate change to Big Tech, The Independent is on the ground when the story is developing. Whether it's investigating the financials of Elon Musk's pro-Trump PAC or producing our latest documentary, 'The A Word', which shines a light on the American women fighting for reproductive rights, we know how important it is to parse out the facts from the messaging.
At such a critical moment in US history, we need reporters on the ground. Your donation allows us to keep sending journalists to speak to both sides of the story.
The Independent is trusted by Americans across the entire political spectrum. And unlike many other quality news outlets, we choose not to lock Americans out of our reporting and analysis with paywalls. We believe quality journalism should be available to everyone, paid for by those who can afford it.
Your support makes all the difference.A new kind of computer intelligence has learned to play dozens of vintage video games without any prior help in how to achieve human-like scoring abilities, scientists said.
The intelligent machine learns by itself from scratch using a trial-and-error approach that is reinforced by the reward of a score in the game. This is fundamentally different to previous game-playing “intelligent” computers, the researchers said.
The system of software algorithms is called Deep Q-network and has learned to play 49 classic Atari games such as Space Invaders and Breakout, but only with the help of information about the pixels on a screen and the scoring method.
The researchers behind the development said that it represents a breakthrough in artificial intelligence capable of learning from scratch without being fed instructions from human experts – the classic method for chess-playing machines such as IBM’s Deep Blue computer.
“This work is the first time anyone has built a single, general learning system that can learn directly from experience to master a wide range of challenging tasks, in this case a set of Atari games, and to perform at or better than human level,” said Demis Hassabis, a former neuroscientist and founder of DeepMind Technologies, which was bought by Google for £400m in 2014.
“It can learn to play dozens of the games straight out of the box. What that means is we don’t pre-program it between each game. All it gets access to is the raw pixel inputs and the game’s score. From there it has to figure out what it controls in the game world, how to get points and how to master the game, just by playing the game,” said Mr Hassabis, a former chess prodigy.
“The ultimate goal here is to build smart, general purpose machines but we’re many decades off from doing that, but I do think this is the first significant rung on the ladder,” he added.
The Deep Q-network played the same game hundreds of times to learn the best way of achieving high scores. In some games it outperformed human players by learning clever tactics such as, for instance, tunnelling through the ends of the wall in Breakout to get the cursor to bounce behind the bricks.
In more than half the games, the system was able to achieve more than 75 per cent of the human scoring ability just by learning by itself through trial and error, according to a study published in the journal Nature.
Deep Blue beat Gary Kasparov, the world champion chess player, in 1997, while IBM’s Watson computer outperformed seasoned players of the quiz show game Jeopardy! in 2011. However, Deep Q works in a fundamentally different way, Mr Hassabis said.
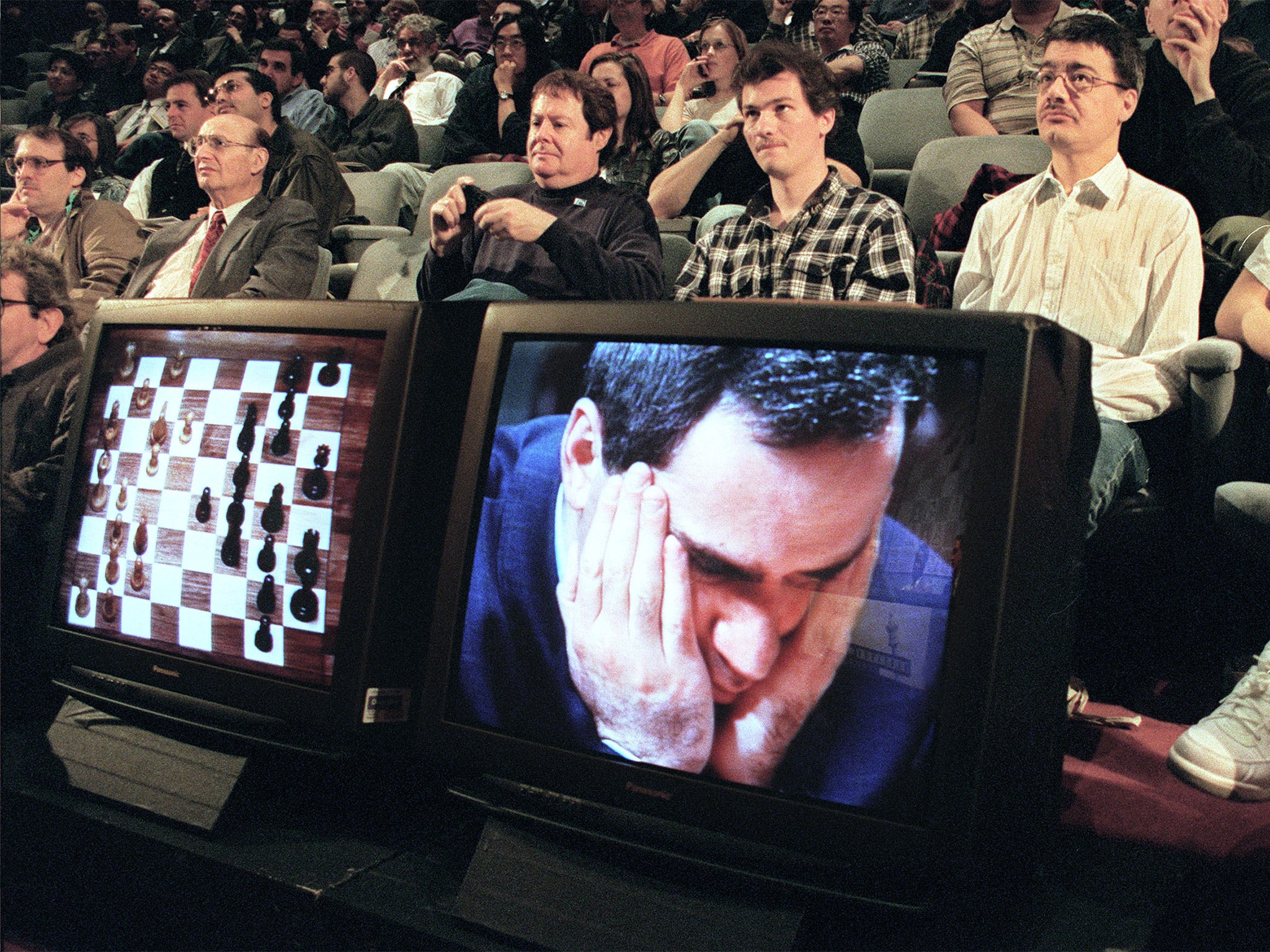
“The key difference between those kinds of algorithms is that they are largely pre-programmed with their abilities,” he explained.
“In the case of Deep Blue it was the team of programmers and chess masters they had on their team that distilled their chess knowledge into a program, and that program effectively executed that without learning anything – and it was that program that was able to beat Gary Kasparov,” he said.
“What we’ve done is to build programs that learn from the ground up. You literally give them a perceptual experience and they learn to do things directly from that perceptual experience from first principles,” Mr Hassabis added.
An advantage of “reinforced learning” rather than “supervised learning” of previous artificial intelligence computers is that the designers and programmers do not need to know the solutions to the problems because the machines themselves will be able to master the task, he said.
“These type of systems are more human-like in the way they learn in the sense that it’s how humans learn. We learn from experiencing the world around us, from our senses and our brains then make models of the world that allow us to make decisions and plan what to do in the world, and that’s exactly the type of system we are trying to design here,” Mr Hassabis said.
What does the creation of Deep Q mean?
A machine with a human-like brainpower is the stuff of nightmares, and even scientists such as Stephen Hawking have warned about the existential threat posed by uncontrolled artificial intelligence.
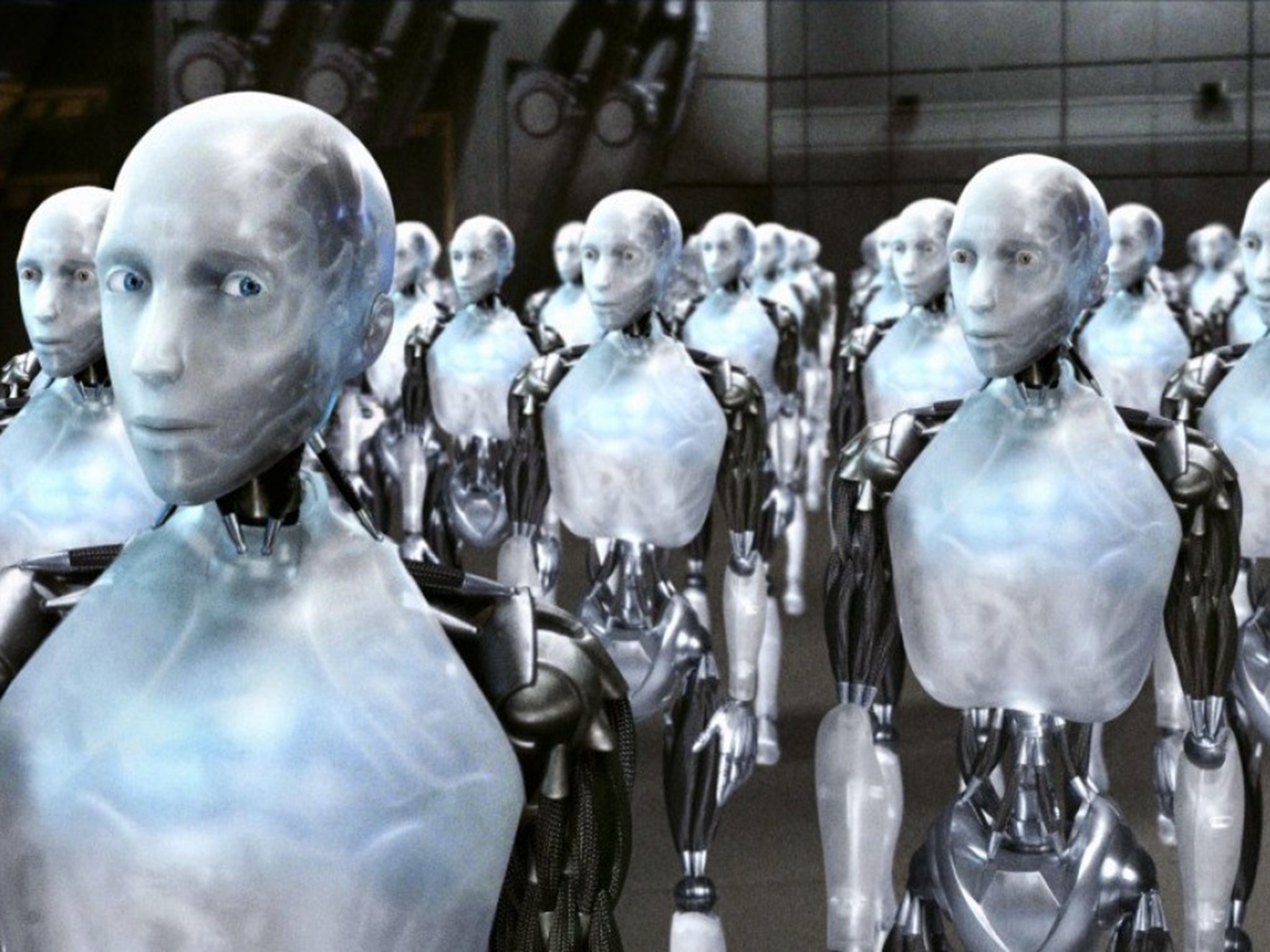
The latest Deep Q-network is far from being able to wield this kind of malign power. However, what makes it interesting, and some might say potentially dangerous, is that it was inspired and built on the neural networks of the human brain.
In particular, the designers of Deep Q compare it to the dopamine reward system of the brain, which is involved in a range of reinforcing behaviours, including drug addiction.
“There is some evidence that humans have a similar system of reinforced learning in the dopamine part of the brain,” said David Silver, one of system’s developers.
“This was one of the motivations for doing our work because humans also similarly learn by trial and error – by observing reward and learning to reinforce those rewards,” he said.
Join our commenting forum
Join thought-provoking conversations, follow other Independent readers and see their replies
Comments